Researchers who have their affiliations with Microsoft and Arizona State University have come up with a technique to detect fake news with the help of “weak social supervision”. According to them, training the fake news detecting AI with the new method can even work when labeled examples aren’t provided as weak social supervision is based on analyzing the user interaction to a post in order to better analyze if the news is misleading or not.
If we go by the statistics given by Pew Research Center, then almost 68 percent of US adults in 2018 had social media as their main source of news. This is rather frightening because of how misinformation spreads easily on social networking sites and a living example of that can be the news regarding COVID-19 pandemic. Although tech giants including Facebook, Google and Twitter are trying their best to detect misleading data, but the thing about fake news is that it continues to change its style which becomes difficult for any AI model to detect efficiently.
Continuing with the study already published in April, the coauthors have further presented a suggestion that weak supervision - a noisy or imprecise source helping data label signals - can result into much better fake news detection without the need for fine-tuning. They also built a framework called as Tri-relationship for Fake News (TiFN) that observes social media users and their connections in the form of “interaction network” only to pick out fake news.
To be precise, interaction network is the link between publishers, news stories, and users. So for an interaction network provided, TiFN then embeds the entities by observing people who choose to interact with like-minded friends. Moreover, upon making the predictions, the framework also takes into consideration the fact that users who are connected may also have shared interest mentioned in the news articles, publishers who are politically-biased can publish fake news and users who have low credibility can also spread fake news.
To test out the effectiveness of TiFN’s weak social supervision model, a Politifact data of 120 true news pieces and 120 verifiable fake pieces were shared in between 23,865 users for validation. The result was also supposed to compete with baseline detectors that only focus on news content and some social interactions. The final outcome, as reported by the researchers showed that TiFN achieved between 75% to 87% accuracy, even with the limitations involved as the role of weak social supervision came into play after only 12 hours of when the news got published.
There was another experiment done on a different Politifact data that was based on 145 true news and 270 fake news pieces having 89,999 comments from 68,523 users on Twitter but now this test involved a separate custom framework called Defend - the one that only picks out news sentences and user comments to state as if the news if fake as per weak supervision signal. Defend was able to score 90% accuracy.
The detection performance came out better when weak social supervision from publisher-bias and user-credibility was used. But when news content, user comment, or the co-attention for news contents and user comments were eliminated, the performance also fell short - which also finally propose that the use of weak social supervision is beneficial.
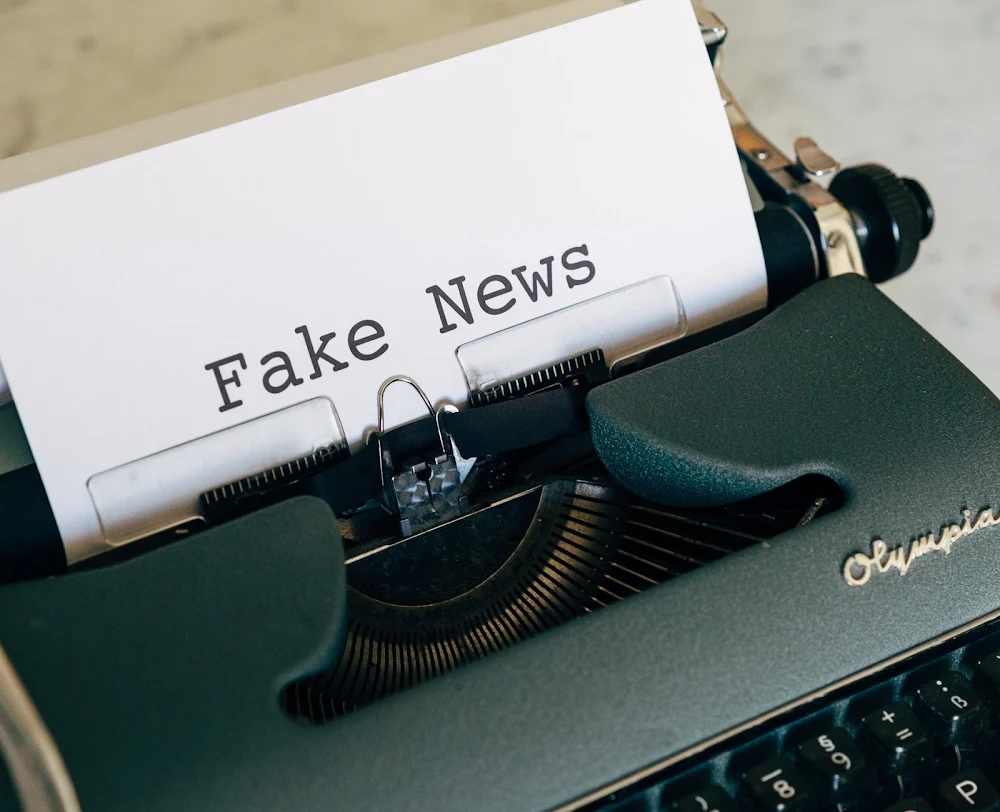
Read next: Microsoft News and MSN Employees Laid Off as Companies Shift to AI Based Operations
If we go by the statistics given by Pew Research Center, then almost 68 percent of US adults in 2018 had social media as their main source of news. This is rather frightening because of how misinformation spreads easily on social networking sites and a living example of that can be the news regarding COVID-19 pandemic. Although tech giants including Facebook, Google and Twitter are trying their best to detect misleading data, but the thing about fake news is that it continues to change its style which becomes difficult for any AI model to detect efficiently.
Continuing with the study already published in April, the coauthors have further presented a suggestion that weak supervision - a noisy or imprecise source helping data label signals - can result into much better fake news detection without the need for fine-tuning. They also built a framework called as Tri-relationship for Fake News (TiFN) that observes social media users and their connections in the form of “interaction network” only to pick out fake news.
To be precise, interaction network is the link between publishers, news stories, and users. So for an interaction network provided, TiFN then embeds the entities by observing people who choose to interact with like-minded friends. Moreover, upon making the predictions, the framework also takes into consideration the fact that users who are connected may also have shared interest mentioned in the news articles, publishers who are politically-biased can publish fake news and users who have low credibility can also spread fake news.
To test out the effectiveness of TiFN’s weak social supervision model, a Politifact data of 120 true news pieces and 120 verifiable fake pieces were shared in between 23,865 users for validation. The result was also supposed to compete with baseline detectors that only focus on news content and some social interactions. The final outcome, as reported by the researchers showed that TiFN achieved between 75% to 87% accuracy, even with the limitations involved as the role of weak social supervision came into play after only 12 hours of when the news got published.
There was another experiment done on a different Politifact data that was based on 145 true news and 270 fake news pieces having 89,999 comments from 68,523 users on Twitter but now this test involved a separate custom framework called Defend - the one that only picks out news sentences and user comments to state as if the news if fake as per weak supervision signal. Defend was able to score 90% accuracy.
The detection performance came out better when weak social supervision from publisher-bias and user-credibility was used. But when news content, user comment, or the co-attention for news contents and user comments were eliminated, the performance also fell short - which also finally propose that the use of weak social supervision is beneficial.
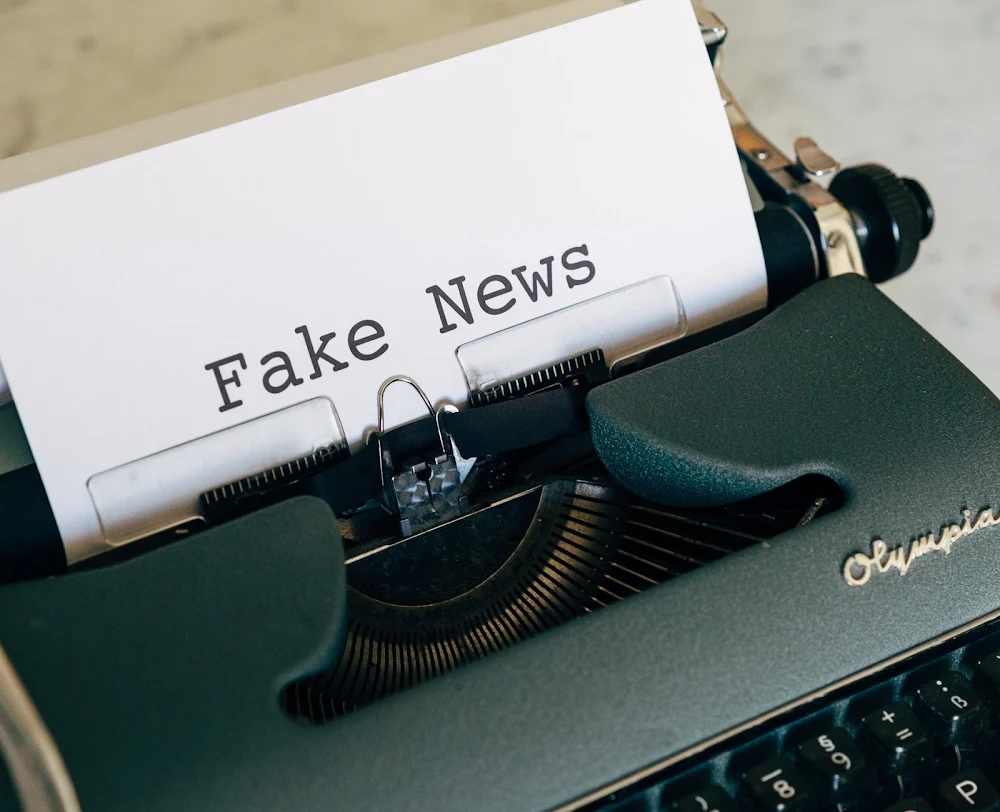
Read next: Microsoft News and MSN Employees Laid Off as Companies Shift to AI Based Operations