Recently, a paper was published on Arxiv by the researchers at Facebook and Hong Kong University of Science and Technology. In this paper, they suggested the use of natural language models as fact-checkers. These models are supposed to be trained on documents of the web and they possess a huge amount of worldly knowledge.
This can be attained by employing a verification classifier model, and when an original claim and a generated claim are given to it, it will determine whether the claim is supported or refuted, or whether the information is correct or incorrect.
Over the years, Facebook has faced numerous issues because of its lenient or non-existing fact-checking systems, and many times the platform has become a source of disinformation and false propaganda. This issue with Facebook has led the platform to lose most of the trust of people all over the world.
According to a survey carried by Zignal Labs, around 86% of American citizens do not fact-check the information they read on social media platforms. More than 61% of people are habitual of sharing, commenting, and liking posts that are recommended by their friends rather than checking for the facts and correctness of the news.
Facebook has been trying hard to redeem its image in the public eye by bringing in more methods and ways to stop misinformation and propaganda, but so far, it has not met with much luck.
Now, with this new research, the co-authors of this paper claim that fact-checking may become more reliable through natural language models. These models will have the ability to memorize information from the world. They will not only make the fact-checking system more efficient; they will also make the process faster by automating the training and verification steps that are conducted by human beings currently. These models will most likely eliminate searches over huge spaces of documents.
These end-to-end fact-checking language models will automatically mask entities like people, things, or places, and tokens or words to recover structures and syntax. This approach basically suggests that factuality often depends on the correctness of entities and the relation between them rather than the phrasing of a claim.
The language model then obtains the top predicted token or word, fills in the masked entity to create an ‘evidence’ sentence. After this, it will use the claim and evidence to obtain entailment features by predicting the ‘truth relationship’ between text pairs.
The researchers conducted these experiments on FEVER (Fact Extraction and VERification) which is a large-scale fact-checking data set containing more than 5.4 million articles by Wikipedia. With their publicly available pre-trained and best performing model BERT, they have managed to achieve 49% accuracy without having to retrieve any document or evidence selection.
However, when they examined BERT’s accuracy, it was found to be pretty short of the system tested against FEVER, which reached 77% accuracy.
This may be because of some limitations of the language models, but the findings suggest that there is a potential of model pretraining through techniques that can store and encode knowledge in a better way. On these grounds, fact-checking systems can be built which can be great at generative questions and answers.
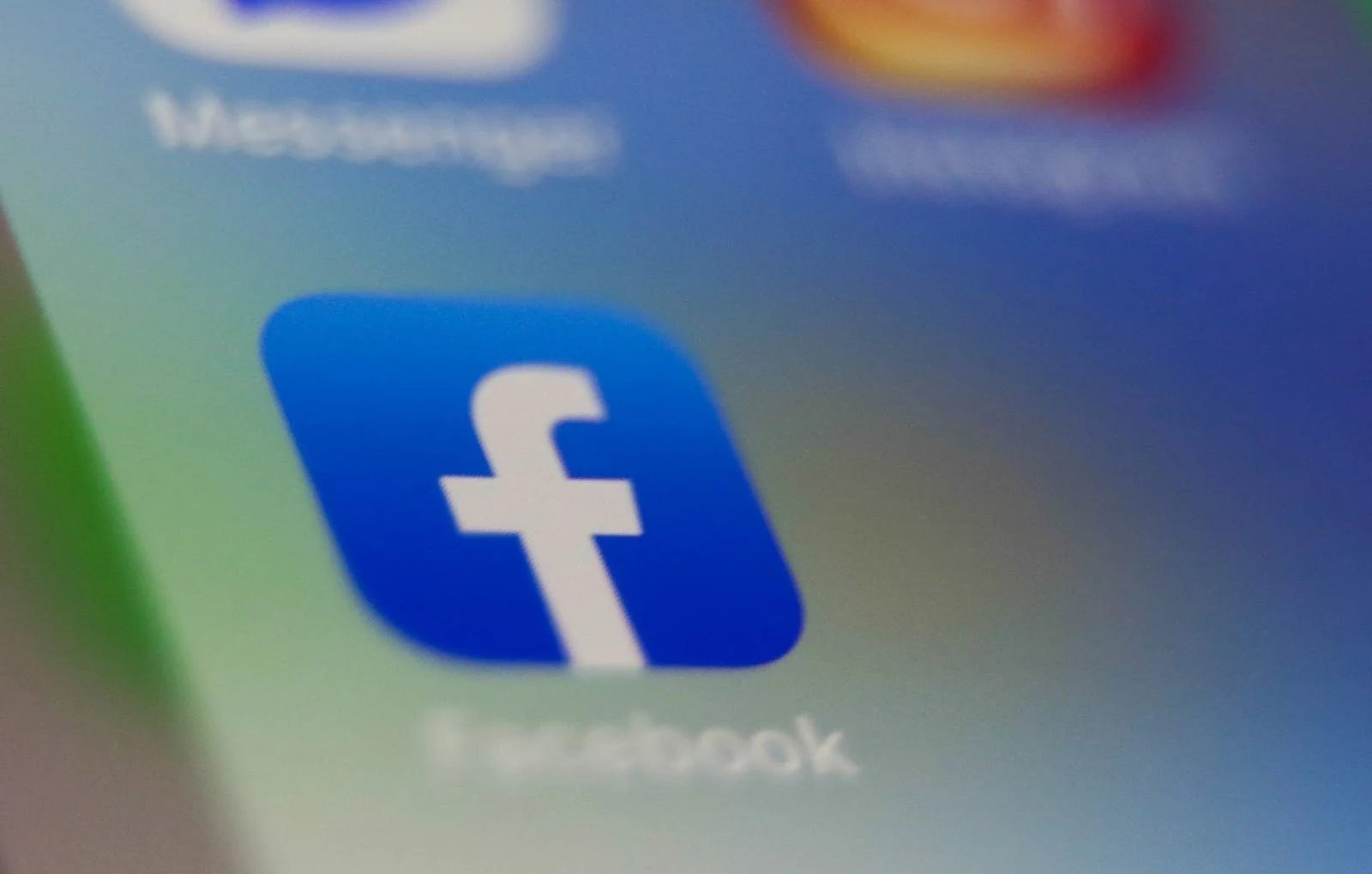
Photo: Jakub Porzycki/NurPhoto via Getty Images
Read next: Facebook is rolling out its News section for all users in the US with inclusions of local news tab
This can be attained by employing a verification classifier model, and when an original claim and a generated claim are given to it, it will determine whether the claim is supported or refuted, or whether the information is correct or incorrect.
Over the years, Facebook has faced numerous issues because of its lenient or non-existing fact-checking systems, and many times the platform has become a source of disinformation and false propaganda. This issue with Facebook has led the platform to lose most of the trust of people all over the world.
According to a survey carried by Zignal Labs, around 86% of American citizens do not fact-check the information they read on social media platforms. More than 61% of people are habitual of sharing, commenting, and liking posts that are recommended by their friends rather than checking for the facts and correctness of the news.
Facebook has been trying hard to redeem its image in the public eye by bringing in more methods and ways to stop misinformation and propaganda, but so far, it has not met with much luck.
Now, with this new research, the co-authors of this paper claim that fact-checking may become more reliable through natural language models. These models will have the ability to memorize information from the world. They will not only make the fact-checking system more efficient; they will also make the process faster by automating the training and verification steps that are conducted by human beings currently. These models will most likely eliminate searches over huge spaces of documents.
These end-to-end fact-checking language models will automatically mask entities like people, things, or places, and tokens or words to recover structures and syntax. This approach basically suggests that factuality often depends on the correctness of entities and the relation between them rather than the phrasing of a claim.
- Also read: On The Footprints Of Google: Facebook Is Now Showing Wikipedia Snippets in Search Results
The language model then obtains the top predicted token or word, fills in the masked entity to create an ‘evidence’ sentence. After this, it will use the claim and evidence to obtain entailment features by predicting the ‘truth relationship’ between text pairs.
The researchers conducted these experiments on FEVER (Fact Extraction and VERification) which is a large-scale fact-checking data set containing more than 5.4 million articles by Wikipedia. With their publicly available pre-trained and best performing model BERT, they have managed to achieve 49% accuracy without having to retrieve any document or evidence selection.
However, when they examined BERT’s accuracy, it was found to be pretty short of the system tested against FEVER, which reached 77% accuracy.
This may be because of some limitations of the language models, but the findings suggest that there is a potential of model pretraining through techniques that can store and encode knowledge in a better way. On these grounds, fact-checking systems can be built which can be great at generative questions and answers.
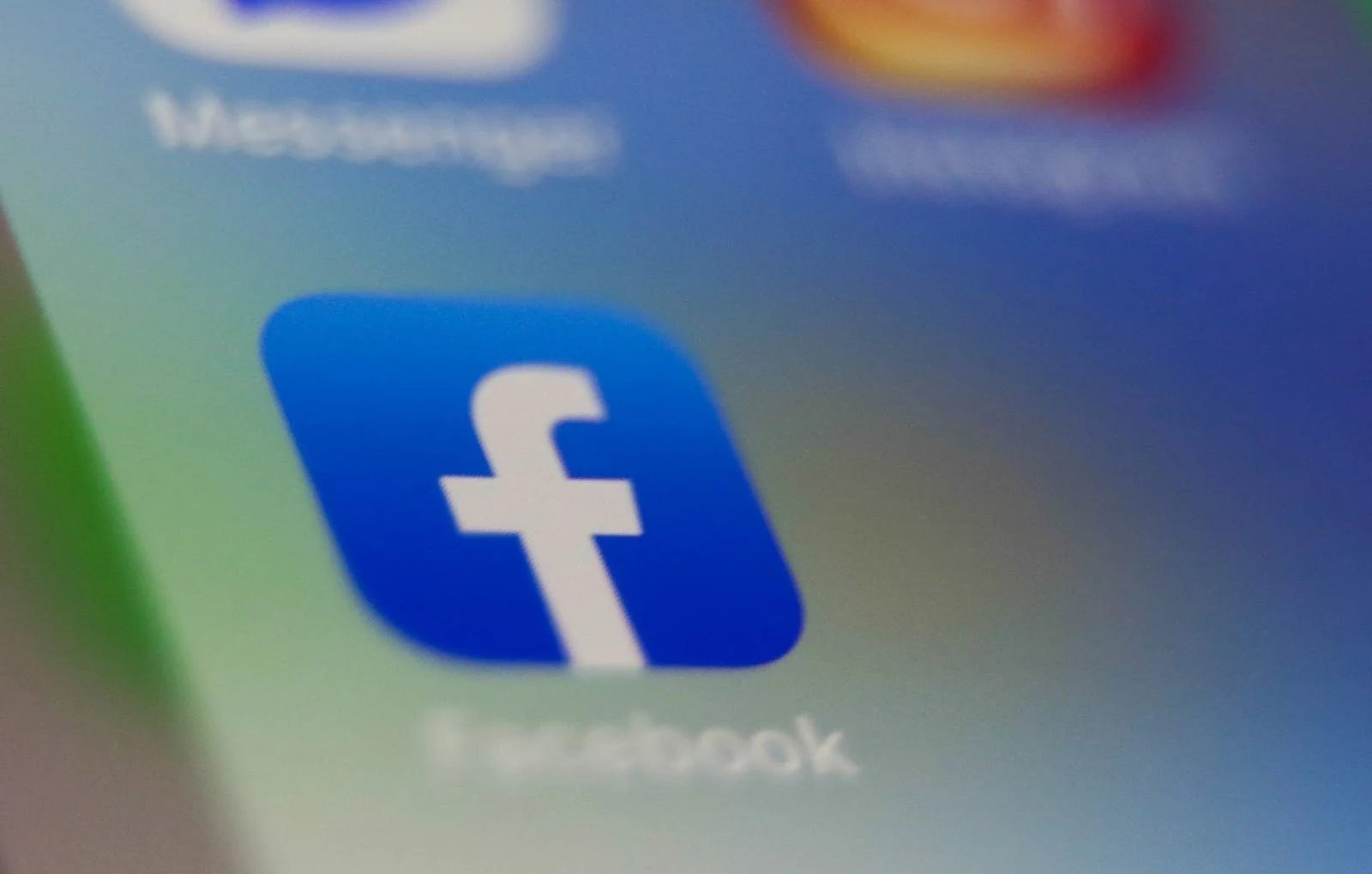
Photo: Jakub Porzycki/NurPhoto via Getty Images
Read next: Facebook is rolling out its News section for all users in the US with inclusions of local news tab